Data ≠ Insight: Improving Metabolomics Data Interpretation
Untargeted biomarker discovery aims to uncover previously unknown factors that associate with biologically relevant changes in human health and disease, such as those related to disease progression or treatment response. Most diseases, while singularly defined pathologically, actually represent diverse groupings of contributing factors and biological pathways. Identifying novel biomarkers can help to elucidate more of these molecular variations so that patients can be stratified and treated based on their unique disease biology.
Metabolomics has emerged as the omics approach that provides the most holistic view of phenotype, and therefore holds significant biomarker discovery potential. Metabolomics profiles small molecules, which read out influences of both genetics and exogenous exposures, such as diet and environment, to gain a systems-wide view of factors that contribute to biological effect. Because of their low molecular weight, small molecules can easily pass through cellular and biological membranes into blood – a readily acquired sample matrix. Advances in mass spectrometry-based technologies have now made it possible to assay thousands of small molecules within a single blood sample at speed, efficiently probing unmapped chemical space to identify new biomarkers of interest.
Not so fast: rapid discovery requires more than analytical speed
While terabytes of spectral data can now be readily captured, the ability to process that data rapidly for accurate, actionable biological insight and biomarker discovery is often still lacking. In fact, a recent poll conducted by Sapient of industry colleagues revealed that data interpretation was their biggest perceived challenge in omics work today. As Sydney Brenner, Nobel laureate and pioneer of molecular biology has said, “we are drowning in a sea of data and starving for knowledge.”
Between the number of samples that can be acquired and analyzed and the number of molecules that can be measured in each sample, the volume of data generated from untargeted small molecule explorations is vast. But how is that output then effectively mined for discoveries?
Data variance must be accounted for
The diversity of the human chemical milieu, combined with the fact that some small molecule levels may vary substantially over time, adds complexity to metabolomics data analysis. Figure 1 shows an example of data generated on Sapient’s rapid liquid chromatography-mass spectrometry (rLC-MS) systems from 50,000 biosamples from healthy, non-diseased individuals. Of the >11,000 small molecules that are captured, about 3,000 are highly stable and represent molecules that are generally tightly regulated under homeostatic control across most humans in the absence of disease. On the other hand, approximately 3,000 small molecules are hypervariable and may significantly vary across similar individuals or even the same individual under different environmental conditions. Because the metabolome is sensitive to both genetic background and environmental stimuli, the interplay between these factors can also confound results.
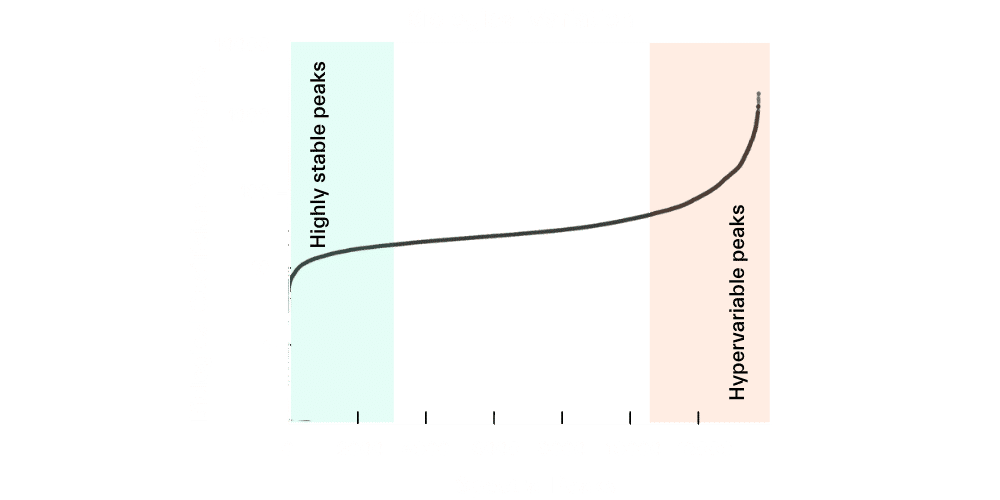
Figure 1. Stable peaks represent small molecules that are tightly regulated with homeostasis of human physiology; hypervariable molecules fluctuate in level within and across individuals over time.
In short, there is no single ‘magic bullet’ to enable more effective data interpretation. It requires optimizations to improve data reproducibility and the accuracy of large dataset processing, and methods that enable us to disentangle endogenous and exogenous sources of variability for greater confidence in results. At Sapient, we think about and address data interpretation challenges in a number of ways, including by (1) ensuring data quality, (2) integrating metabolomics data with other data sets, and (3) leveraging our expansive Human Biology Database to confirm findings at a population level.
(1) Bridging the gap to insight: data quality is the first step
Sophisticated mass spectrometry methods backed by rigorous quality control (Qc) protocols are the foundation for delivering high quality metabolomics analyses and reproducible data. Without stringent standards to mitigate process-based sources of variation, the data and resulting statistical findings can end up biased. Sapient deploys multiple levels of Qc to ensure optimal, consistent performance of our rLC-MS systems for untargeted discovery, including system suitability Qc, in-plate pooled plasma bracket Qc, and isotopically labeled internal standard Qc.
However, even when spectral data is of high technical quality, processing it for insight requires identification and isolation of true spectral features from random, false noise peaks. Many conventional peak detection workflows still rely on inexact peak selection algorithms and time-consuming visual inspection of data. This can lead to the inclusion of many false positive peaks while at the same time may overlook true signals. This solution is also not scalable when the number of spectral peaks is high or sample size is large, as is often the case with untargeted metabolomic analyses.
To improve data interpretation, new strategies have been developed that increase both the capacity and fidelity of large-scale metabolomics data processing. The application of machine learning (ML) in this area, for example, is showing great promise to improve peak detection. Sapient has developed our own ML-based approaches that are able to remove up to 90% of false peaks from complex, untargeted LC-MS data sets without reducing true positive signals.
(2) Reading the right story: interpreting what the data tells us
Having the right, robust data in hand gives data science teams the ability to more accurately interpret biological insights. Data can be confidently interrogated to uncover key biomarkers that relate specifically to biological processes, disease progression, or drug responsiveness. The key to making sense of the story that biomarkers are telling, however, is to understand the story has co-authors.
Small molecule biomarkers provide powerful insights into disease chemistry and drug pharmacology, but often correlate with other biomolecules that also influence observed biochemical effects. Accurate metabolomics data interpretation requires integration with other omics data, clinical data, and human health metadata to reveal the most comprehensive view of underlying disease biology and drug effects. Sapient’s biocomputational approach amalgamates an array of high dimensional data – from genomics, proteomics, preclinical models, human biology insights, and clinical outcomes – to account for the interplay between factors and their impact on findings.
Because small molecule biomarkers are often conserved from preclinical to clinical studies, it can also be valuable to assess them in multiple model systems (Figure 2). For example, if we find a biomarker change in humans indicating drug effect, we can evaluate that same biomarker in a cellular study – where external variables can be closely controlled – to confirm if similar effect occurs regardless of environmental influences.
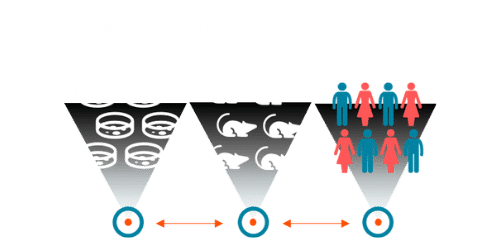
Figure 2. Small molecule biomarkers are conserved across model systems, providing a unique advantage to assess biomarker changes with and without environmental variability for added confidence in human study findings.
(3) Confirmation of findings: cross-validation to build confidence in biological insights
Sapient is able to take insight confirmation a step further by leveraging our Human Biology Database with data from biosamples from more than 100,000 individuals that have already been run on our rLC-MS platform. This data is linked to longitudinal tracking information, with these individuals having been clinically followed for a range of 10-30 years with information on their demographics, lifestyle factors, medication regimens, and more.
This allows us to understand a small molecule biomarker’s stability over time, and how it is influenced by endogenous and exogenous factors such as diet, exercise, toxicants, other drugs, as well as internal organs and the microbiome (Figure 3). Importantly, we also have data from dense sampling of individuals over much shorter periods of time including down to the course of a day, which reveal important insights about how these molecules change with circadian rhythm, fasting/feeding cycles, and in response to different dietary challenges.
Our database can be readily tapped to confirm biomarker findings in a large-scale, population-based cohort, providing the statistical power to validate the specificity and sensitivity of biomarkers identified in the data interpretation phase.
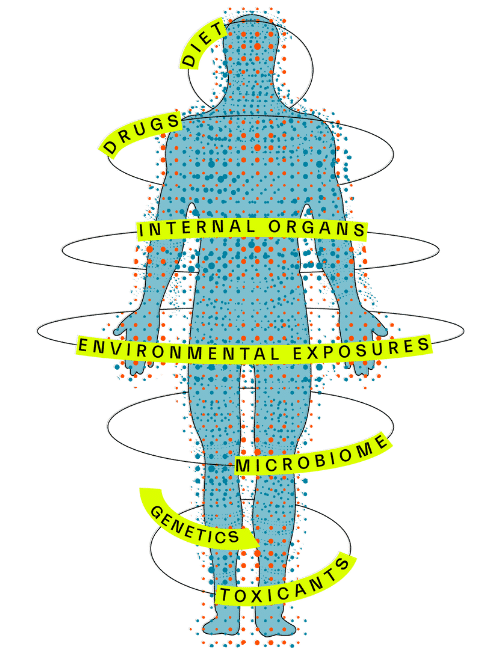
Figure 3. Sapient’s Human Biology Database includes longitudinal tracking information that elucidates how endogenous and exogenous factors influence small molecule biomarker levels over time.
Extracting meaningful insight requires deep knowledge
It is imperative to deploy the right approaches to generate, handle, and extract meaningful data from large, untargeted metabolomics analyses, so that biocomputational analysis yields meaningful insight. The team managing these processes must have the deep statistical and machine learning skills as well as biological knowledge to accurately identify and interpret biomarker findings that will answer unknowns about human biology and advance our ability to align patients, disease, and therapies for greater drug development success and better health outcomes.
Interested in speaking to the Sapient data science team? Schedule a time to talk here or by emailing discover@sapient.bio.