Article | March 29, 2024
Subtyping, Predicting, and Treating Chronic Kidney Disease: Finding New Paths with Nontargeted Biomarker Discovery
Chronic kidney disease (CKD) is a widespread and often silent condition affecting millions worldwide, with its prevalence steadily rising. CKD can be caused by a myriad of factors, ranging from underlying genetics to environment and lifestyle. Prolonged high blood sugar levels in diabetes and persistent elevated blood pressure in hypertension, for example, exert chronic stress on the kidneys, impairing their function over time. Other medical conditions such as autoimmune diseases, polycystic kidney disease, and recurrent kidney infections can also lead to CKD development, as can certain medications, smoking, alcohol consumption, and diet.
This multifaceted disease presents significant challenges in both diagnosis and management, necessitating a more nuanced approach for effective treatment. Existing classification systems often subtype CKD from mild (Stage 1) to severe (Stage 5), usually based on factors such as estimated glomerular filtration rate (eGFR) and urine protein levels. While these measures have long been used to diagnose and assess disease severity, they are known to be imprecise. Further, these stages are based on the degree of kidney damage that has already occurred rather than risk factors, particularly for early- vs. late-onset disease.
The rise of next-generation bioanalytical technologies, such as high throughput mass spectrometry, has afforded us an invaluable opportunity to better understand the intricacies of CKD. These systems enable nontargeted biomarker discovery approaches to probe a broader range of dynamic biomarkers – including metabolites, lipids, and proteins – that read out both endogenous and exogenous factors influencing disease development over time. Instead of relying on only known, well-established measures of CKD severity, we can now ‘open the aperture’ with a more exploratory, non-biased approach to capture other biologically relevant signals that could potentially be better predictive and prognostic biomarkers for patient stratification. Advances in big data infrastructures are also enabling population-based, longitudinal studies, where we can collect and compare these comprehensive sample measures across tens of thousands of patients and timepoints to identify trends and biomarker correlations we would not have identified otherwise – including discovery of early biomarkers of disease.
In this article we will discuss how dynamic biomarkers discovered via nontargeted approaches can provide immense value in understanding CKD biology, assessing disease progression, and helping to subtype patients to manage and treat CKD more effectively.
Navigating the heterogeneity in CKD populations
CKD, like many chronic diseases, encompasses a diverse array of etiologies, clinical presentations, and trajectories. Clinically, CKD can present with a spectrum of symptoms ranging from asymptomatic early stages to advanced stages characterized by complications such as fluid overload, electrolyte imbalances, anemia, and cardiovascular disease. CKD disease progression also varies widely among individuals, with some experiencing a slow decline in kidney function over many years, and others progressing rapidly to end-stage kidney disease. This immense heterogeneity has made it challenging to identify meaningful biomarkers using more traditional, targeted approaches.
While several genes have been associated with CKD, studies show that the majority of disease risk for chronic diseases is not attributable to genetics alone. Because the human genome is largely static from conception, genomics data is limited in its utility to account for changes in health status over time. An understanding of how diet, medication regimen, exercise levels, and other lifestyle factors interact with genetics and influence disease is needed to decipher the complexities of CKD.
Large-scale profiling of metabolite, lipids, and proteins can capture the non-genetic factors that influence CKD development and progression, helping to characterize the full spectrum of disease. By analyzing the varied metabolite and protein biomarker profiles of CKD, for example, there is potential to better stratify patients by different disease subtypes – by the specific ‘pathway’ by which they went from a normal to a disease state – and thereby provide more appropriate and personalized care. Small molecule and protein biomarkers also enable researchers to better understand and identify potential CKD risk factors beyond genetics and family history, to ultimately empower doctors to intervene earlier and halt disease progression before it advances to the point that dialysis or a kidney transplant is required.
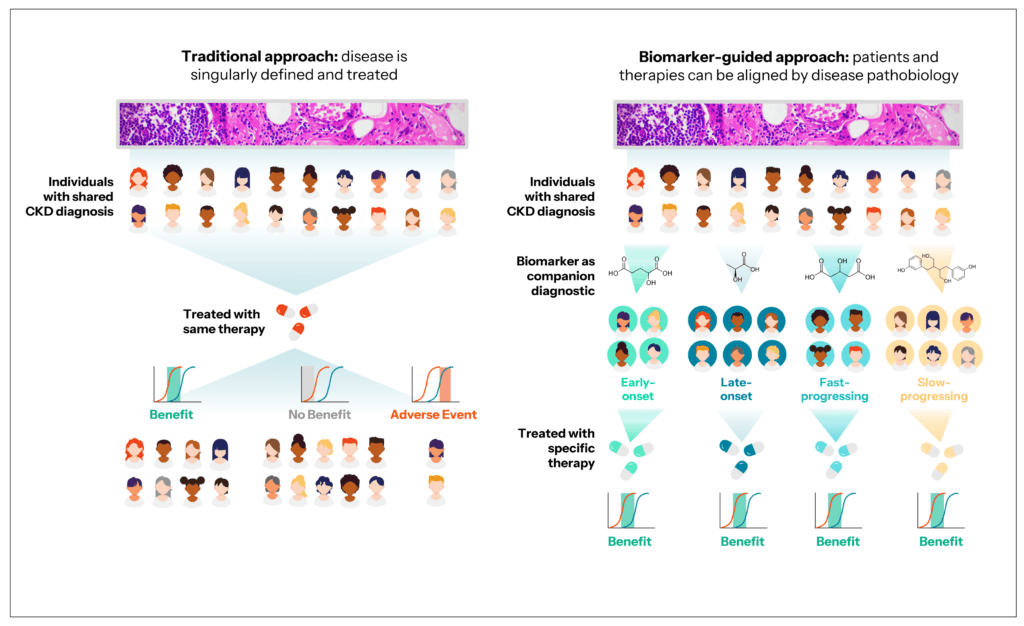
Discovering new prognostic biomarkers to track CKD progression
While eGFR is a widely used and important measure for assessing kidney function and tracking CKD progression, it does have its limitations and challenges. For example, eGFR estimation equations are less precise at higher levels of kidney function. As a result, small changes in eGFR within this range may not be accurately detected, potentially delaying the identification of early-stage kidney disease.
Metabolite and protein biomarkers offer several key advantages that can complement eGFR values to better assess disease progression. Perhaps one of their biggest advantages in the context of CKD is that they are readily measured in fluids, such as blood and urine, which are already routinely collected for kidney analyses. Because of this, biomarkers can be easily tracked across multiple timepoints, over weeks, months, and years, without having to conduct invasive biopsies or imaging.
The high throughput capacity now afforded by next-generation mass spectrometry also means that these multi-omics measures can be efficiently collected in thousands – or even tens of thousands – of samples, providing the scale and statistical power to understand how CKD biomarkers behave across diverse populations. For example, at Sapient we have used our rLC-MS systems to build a longitudinal Human Biology Database with this purpose in mind. It is comprised of data from over 100,000 biosamples with paired phenotypic measures from individuals around the world – including several thousand people with renal diseases of different subtypes, such as fast progressors and those with rare kidney diseases. In a large number of these individuals, we also have paired genetics information, allowing us to perform integrative analyses that bridge genotype and phenotype and evaluate biomarker associations against different hypotheses.
It is clear that dynamic biomarkers have a key role to play in advancing our understanding of CKD to better diagnose, treat, and manage the growing population of patients afflicted by this disease. The value of these biomarkers lies in their ability to further subtype CKD and identify potential risk factors beyond genetics, to guide more personalized treatment strategies and improved patient outcomes.
Advancements in bioanalytical technologies are enabling nontargeted approaches to broadly screen the metabolome, lipidome, and proteome beyond ‘known’ biomarkers to identify novel measures that can guide patient stratification, prognosis, and therapeutic development. These methods are expected to accelerate the discovery and implementation of new biomarkers into the CKD diagnosis and treatment paradigm.